In my last post, I talked about the benefits of an opinionated platform. An opinionated platform allows your engineers to focus on things that matter to your business, such as shipping and improving customer-facing products and services. This is in contrast to engineers spending substantial time on non-differentiating work like platform infrastructure. Rather than infrastructure architecture, developers can focus more on the product architecture. Konfig is an opinionated platform which provides two key value drivers: 1) reducing the investment and total cost of ownership needed to have an enterprise cloud platform and 2) minimizing the time to deliver new software products.
Konfig provides an out-of-the-box, enterprise-grade platform which is built with security and governance at its heart. Building this type of platform normally requires a sizable team of platform engineers which takes constant care, maintenance, and ongoing investment. With Konfig, we can now reallocate these resources to higher-value work, and we only need a small team to manage resource templates used by developers and implement business-specific components. It enables this small team to provide a robust platform within their company with organizational standards and opinions built in. This, in turn, allows an organization’s developers to self-service with a high degree of autonomy while ensuring they work within the bounds of our organization’s standards.
For many organizations, bringing a new software product to market can be a monumental undertaking. Even when the code is written, it can take some companies six months to a year just to get the system to production. Konfig reduces this sunk cost by accelerating the time-to-production. This is possible because it provides an opinionated platform that solves many of the common problems involved with building software in a way that codifies industry best practices. Konfig’s approach encourages deploying to production-like environments from day-1, something we call Deployment-Driven Development.
So what are Konfig’s opinions? What is the motivation behind each of them? And does an opinionated platform mean an organization is constrained or locked in as is often the case with a PaaS? Let’s explore each of these questions.
Opinions and their benefits
GitLab and GCP
Perhaps the most obvious of Konfig’s opinions is that it is centered around Google Cloud Platform and GitLab. While we are actively exploring support for GitHub and AWS, we chose to start with building a white-glove experience around GCP and GitLab for a few reasons.
First, GCP has best-in-class serverless offerings and managed services which lend themselves well to Konfig’s model. This is something we’ve written about extensively before. Services like Cloud Run, Google Kubernetes Engine (GKE), BigQuery, Firestore, and Dataflow are truly differentiators for Google Cloud.
Second, GCP’s Config Connector operator provides, we argue, a better alternative to Terraform for managing infrastructure. We’ll discuss this in more detail later.
Third, we believe GitLab’s CI/CD system is better designed than GitHub Actions. This is something worthy of its own blog post, but it’s a key factor in providing a platform that is both secure and has a great developer experience.
Lastly, GitLab uses a hierarchical structure with groups, subgroups, and projects which maps perfectly to Konfig’s own control plane, platform, domain hierarchy as well as GCP’s resource hierarchy with organizations, folders, and projects. This is a critical component in how Konfig manages governance.
The Konfig model works with any combination of cloud platform and DevOps tooling. We just chose to start with GCP and GitLab because they work so well together. With Konfig, they almost feel as if they are natively integrated.
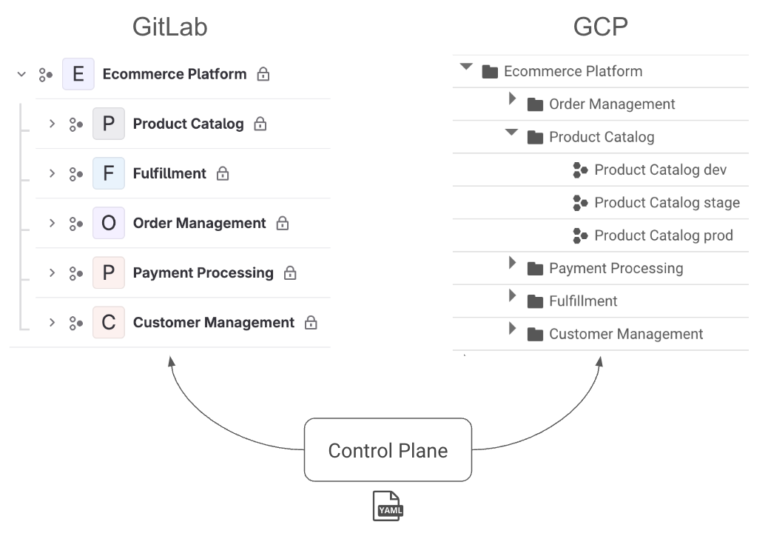
Service-oriented architecture and domain-driven design
Konfig has a notion of platforms and domains. A platform is intended to map to a coarse-grained organizational boundary such as a product line or business unit. Platforms are further subdivided into domains, which are groupings of related services. This is loosely borrowed from the concept of domain-driven design. While Konfig does not take a particularly strong stance on DDD or how you structure workloads, it does encourage the use of APIs to connect services versus sharing databases between them.
This is a best practice that Konfig embraces because it reduces coupling which makes it easier to evolve services independently. A key aspect of this grouping will make certain tasks harder (though not necessarily impossible), on purpose, such as sharing a database between domains. It also promotes more durable teams who own different parts of a system, which is an organizational best practice we routinely encourage with our clients.
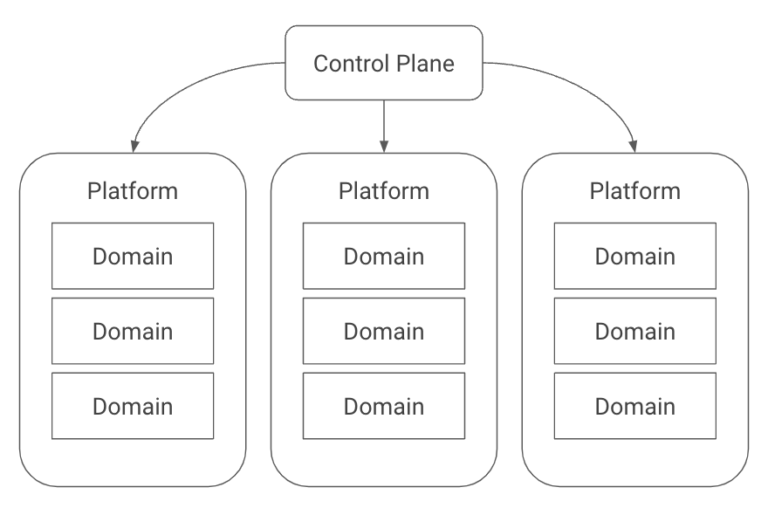
Structuring GitLab and GCP
Konfig maintains a consistent structure between GitLab and GCP based around the control plane, platform, domain hierarchy. Control planes, platforms, and domains are all declaratively defined in YAML. This hierarchy is central to Konfig because it allows it to enforce best practices for access management and cloud governance. It also provides powerful cost visibility because we can easily see the cloud spend and forecasted spend for platforms and domains. This means there is a rigid opinionation to the tiered structuring of folders and projects in GCP and subgroups and projects in GitLab.
In GCP, a control plane maps to a folder within your GCP organization (either specified by the user during setup or created by the Konfig CLI). Within this folder, there is a control plane project which houses the control plane Kubernetes cluster and a folder for each platform. Within each platform folder, there are folders for each domain which contain a project for each environment (e.g. dev, stage, and prod).
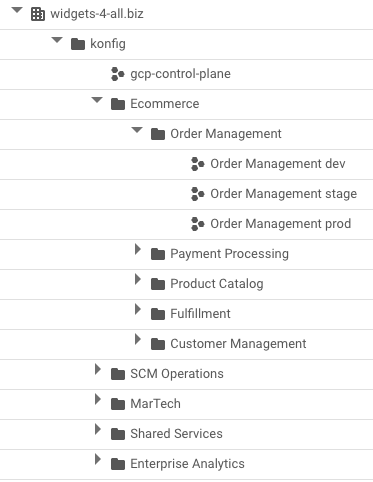
In GitLab, a control plane maps to a subgroup within your organization’s top-level group (again, either specified by the user during setup or created by the CLI). Within this subgroup, there is a control plane project which houses the definitions for the control plane itself as well as the platforms and domains it manages. In addition to the control plane project, the control plane subgroup contains a child subgroup for each platform. Like the GCP structure, these platform subgroups in turn contain subgroups for each of the platform’s domains. It’s in these domain subgroups that our actual workload projects go. Konfig provides a GitLab template for creating new workload projects that includes a fully functional CI/CD pipeline and workload definition for configuring service settings and infrastructure resources.
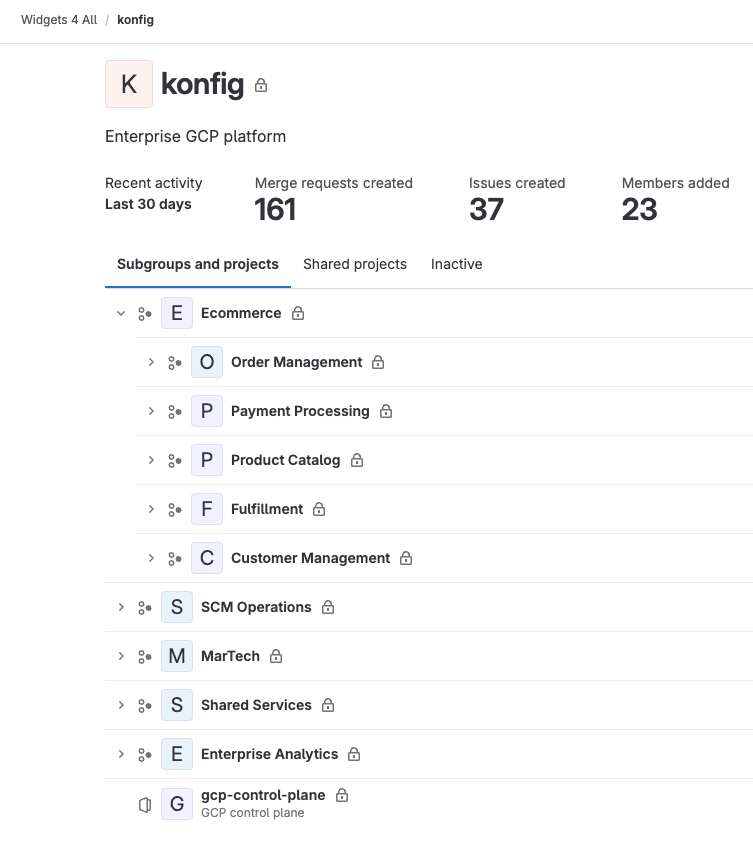
This structure is critical because it allows Konfig to manage access and permissioning for both users as well as service accounts. This enables the platform to enforce strong isolation and security boundaries. The hierarchy also allows us to cascade permissions and governance cleanly.
Group-based access management
Konfig leverages groups to manage permissioning. Groups are managed using a customer’s identity provider, such as Google Cloud Identity or Microsoft Entra ID (formerly Azure AD). These groups are then synced into GitLab using SAML group links and into GCP with Cloud Identity (if using an external IdP). In the control plane, platform, and domain YAML definitions, we can specify what GitLab and GCP permissions a group should have from a single configuration source.
This opinionated model provides a single source of truth for both identity (by relying on a customer’s existing IdP) and access management (via Konfig’s YAML definitions). We’ve often seen organizations assign roles to individual users which is an anti-pattern, so Konfig relies on groups as a best practice. This model also lets us apply SDLC practices to access management.
apiVersion: konfig.realkinetic.com/v1beta1
kind: Domain
metadata:
name: order-management
namespace: konfig-control-plane
labels:
konfig.realkinetic.com/platform: ecommerce
spec:
domainName: Order Management
gcp:
createProjects: true
enableWorkloadIdentity: true
envs: [dev, stage, prod]
manageFolder: true
gitlab:
manageCIVars: true
manageGroup: true
groups:
dev: [order-management-devs@widgets-4-all.biz]
maintainer: [order-management-maintainers@widgets-4-all.biz]
owner: [sre-admins@widgets-4-all.biz]
Example domain.yaml showing group permissions
Workload identity and least-privilege access
One of the benefits of Konfig is that it automatically manages IAM for workloads. This means you just specify what resources your application needs, such as databases, storage buckets, or caches, and it not only provisions those resources but also configures the application’s service account to have the minimal set of permissions needed to access them for the role specified.
In Konfig, every workload gets a dedicated service account. This is a best practice that ensures we don’t have overly broad access defined for services. Often what happens otherwise is service accounts get reused across applications, resulting in workload identities that accrue more and more roles. Another common anti-pattern is using the Compute Engine default service account which many GCP services use if a service account is not specified. This service account usually has the Editor role, which is a privileged role that grants broad access. Konfig disables this default service account altogether, preventing this from happening.
Decoupling IAM for developers, CI/CD, control planes, and workloads
There are four main groups of identities in Konfig: users, CI/CD pipelines, control planes, and workloads. Konfig takes the position that human users should generally not require elevated permissions. Similarly, all modifications to environments should occur via CI/CD pipelines rather than manually or through “ClickOps.” For this reason, Konfig enforces strong separation of developer user accounts, CI/CD service accounts, control plane service accounts, and workload service accounts.
Earlier we saw how Konfig relies on group-based access management rather than assigning roles to individual users. This provides a more uniform approach to access management. These roles provide a limited set of permissions. Instead, developers interact with Konfig through GitLab pipelines. Note, however, that the “owner” group permission, which is illustrated in the example domain.yaml above, provides break-glass access that can be set at the domain, platform, and control plane level to support situations that require emergency remediation.
Konfig maps GCP service accounts to CI/CD pipelines in GitLab using Workload Identity Federation. The service accounts used by the CI/CD system have limited permissions that are scoped by GCP IAM and Kubernetes RBAC. This means that a pipeline for a specific workload in a domain can only apply modifications to its own control plane namespace. This greatly reduces the blast radius of a compromised GitLab credential and also prevents teams from modifying environments that they don’t own.
Additionally, because Konfig relies on Workload Identity Federation, there are no long-lived credentials to begin with. Workload Identity Federation uses OpenID Connect to allow a GitLab pipeline to authenticate with GCP and use a short-lived token to act as a GCP service account. This is in contrast to using service account keys for authenticating between GitLab and GCP, which is a security anti-pattern because it involves long-lived credentials that often do not get rotated. These keys are a common source of security breaches. And because the Konfig control plane is responsible for orchestrating resources, this CI/CD service account needs a very minimal set of permissions. Basically, it just needs permissions to apply Konfig definitions to its control plane namespace. The control plane handles the actual heavy lifting from that point on.
Each domain-environment pair gets its own namespace in the Konfig control plane. This namespace has its own service account that is scoped only to the GCP project associated with this domain environment. This allows the control plane to provision workloads and resources within a domain while having strong isolation between different domains and different environments.
We already discussed how Konfig manages IAM for workloads and implements least-privilege access. It’s important to note that, like the CI/CD service accounts, these workload service accounts have no keys associated with them and thus are never exposed to humans or to the CI/CD system. This means they are fully decoupled and easier to audit and monitor.
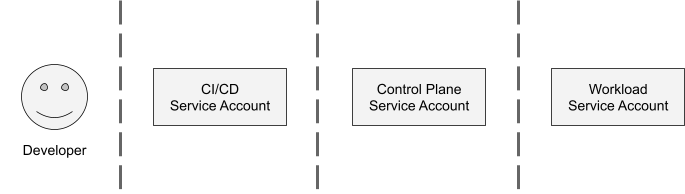
GitOps, branching, and release strategies
Konfig uses a GitOps model for managing platforms, domains, workloads, and infrastructure resource templates. These constructs are defined in YAML and deployed or promoted using Git-based workflows like merging a branch into main or tagging a release. This model is a best practice that provides a declarative single source of truth for our infrastructure (which is normally referred to as Infrastructure as Code or IaC), our GitLab and GCP implementations, and our organizational standards (by way of resource templates). For instance, we saw earlier how these declarative configurations are used to manage permissions in GitLab and GCP. This allows us to apply the same SDLC we use for application source code to our enterprise platform. This more comprehensive approach to managing infrastructure, source control, CI/CD, and cloud environment is something we call Platform as Code.
Konfig promotes a trunk-based development workflow with merge requests and code reviews. Releases are done by creating a tag. This GitOps model provides a clear audit trail and approval flow that most developers are already familiar with. This not only lends itself to providing a better developer experience but also a strong governance story. Infrastructure configuration is treated as data stored in source control. This makes it easy to backup and restore, but we’ve also chosen a format that is widely supported and makes writing custom tooling or integrating with existing tools easy.
Image promotion and single container artifact
Workload repositories can only contain a single deployable artifact. This means monorepos are not supported in Konfig, and repositories may only have a single Dockerfile that gets built and deployed. It also means workloads need to be containerized. This allows Konfig to make certain assumptions about CI/CD and SDLC that further improve the developer experience, security, and governance.
A problem we see regularly at companies is images getting rebuilt for different environments. Konfig’s image promotion model ensures the images used for testing are what is deployed to production without rebuilding containers or copying artifacts from development environments. The use of releases and environments in GitLab ensures there is a clear auditing and tracking of artifacts so that you know exactly what is deployed, by whom, and when.
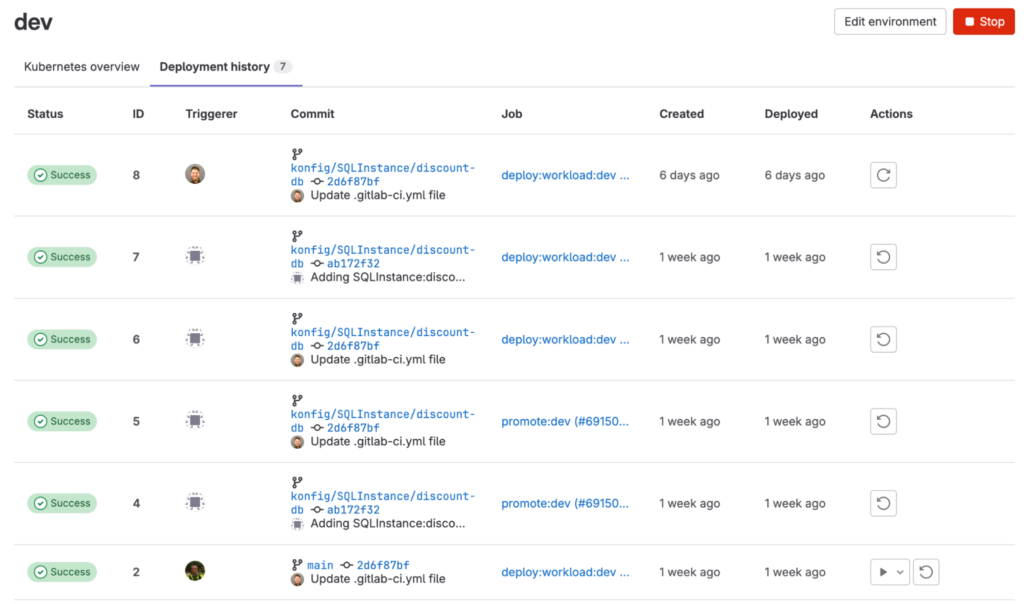
Managed services and serverless over other options when possible
We’ve spoken at length about the benefits of serverless and how, for many businesses, it may be a better fit than Kubernetes. Previously, I mentioned that one of the reasons we chose GCP initially to build an enterprise-ready platform was because of its emphasis on serverless and managed services. In particular, services like Cloud Run, GKE, and Dataflow are truly industry-leading container platforms. For this reason, Konfig supports these runtimes natively. We recommend Cloud Run as the default workload runtime but provide GKE as a supported engine for cases where Cloud Run is just not a good fit. Dataflow provides a fully managed execution environment for unified batch and stream data processing.
Leveraging managed services and serverless greatly reduces operational burden, improves security posture, allows developers to focus on product and feature development, and reduces production lead times. By supporting a smaller set of services, we can provide a great developer experience and security posture by automatically configuring service account permissions, autowiring environment variables in application containers, managing secrets, and a number of other benefits. Reducing options also simplifies architecture, improves maintainability and supportability, and reduces infrastructure sprawl. We’ve said before, we believe organizations should invest their engineers’ creativity and time into differentiating their customer-facing products and services, not infrastructure and other non-differentiating work.
This is similar in nature to PaaS, but where Konfig differs is it provides an “escape hatch.” That is to say, it provides well-supported paths for both working around the platform’s opinions and constraints and for moving off of the platform if needed. I’ll touch on these a bit later.
Resource templates over bespoke configurations
Infrastructure as code is often quite complicated because infrastructure is complicated. If you’ve ever worked with a large Terraform configuration you’ve probably experienced the challenges and pain points. It can be tedious to maintain and every implementation of it is different from company to company or even team to team. Konfig takes a different approach that provides an improved developer experience and stronger governance model. Workload definitions specify important metadata about a service such as the runtime engine, CPU and memory settings, infrastructure resources, and dependencies on other services. These definitions provide a declarative and holistic view of a workload that sits alongside the source code and follows the same SDLC.
Below is a simple workload.yaml for a service with three infrastructure resources, a Cloud Storage bucket, a Cloud SQL database, and a Pub/Sub topic. If you recall, the Konfig control plane will handle provisioning these resources and configuring the service account with properly scoped roles. It will also inject environment variables into the container so that the service can “discover” these resources at runtime.
apiVersion: konfig.realkinetic.com/v1beta1
kind: Workload
metadata:
name: payment-api
spec:
region: us-central1
runtime:
kind: RunService
parameters:
template:
containers:
- image: payment-api
resources:
- kind: StorageBucket
name: receipts
- kind: SQLInstance
name: payment-db
- kind: PubSubTopic
name: payment-authorized-events
Example workload.yaml showing resource dependencies
As you can imagine, there’s a lot more to configuring these resources than simply specifying their name. This is where Konfig’s notion of resource templates comes into play. Konfig relies on resource templates to abstract the complexity of configuring cloud resources and provide a means to implement and enforce organizational standards. For instance, we might enforce a specific version of PostgreSQL, high availability mode, and customer-managed encryption keys. For non-production environments, we may use a non-HA configuration to reduce costs.
This model allows a platform engineering or SRE team to centrally manage default or required configurations for resources. Now organizations can enforce a “golden path” or a standardized way of building something within their organization. Rather than relying on external policy scanners like Checkov that work reactively, we can build our policies directly into the platform and hide most of the complexity from developers, allowing them to focus on what matters: product and feature work. An organization can choose the right balance between autonomy and standardization for their unique situation, and we can eliminate infrastructure and architecture fragmentation.
apiVersion: sql.cnrm.cloud.google.com/v1beta1
kind: SQLInstance
metadata:
name: high-availability
namespace: konfig-templates
labels:
annotations:
konfig.realkinetic.com/extra-fields: "settings.tier,settings.diskSize"
spec:
databaseVersion: "POSTGRES_15"
settings:
tier: "db-custom-1-3840"
diskSize: 25
availabilityType: REGIONAL
diskType: PD_SSD
backupConfiguration:
binaryLogEnabled: true
enabled: true
Example resource template for Cloud SQL
API ingress and path-based routing
Standardizing API ingress and routing is another key part of reducing architecture fragmentation and improving developer productivity. Konfig takes an opinionated stance on how workloads interact with each other and how external traffic interacts with a workload. By default, workloads are only accessible to other workloads in the same domain. However, we can also expose workloads to other workloads in the same platform or even across platforms if they are within the same control plane. Lastly, we can expose a workload to external traffic from the internet or from other control planes.
Konfig manages load balancers to make this ingress seamless and straightforward. It also utilizes path-based routing that maps to the platform, domain, workload hierarchy to provide a clean way of exposing APIs. Path-based routing is a best practice we promote because, compared to host-based routing, there’s less infrastructure to maintain, it removes cross-origin resource sharing (CORS) as a concern, and there are significantly fewer DNS records involved. A common challenge for SaaS companies is getting customers to whitelist hostnames. This is often a major headache for enterprise customers where whitelisting hostnames can be difficult. Path-based routing eliminates this problem by exposing services under the same domain.
Config Connector for IaC rather than Terraform
As mentioned earlier, one of the reasons we chose GCP as the initial cloud platform supported in Konfig is Config Connector. Config Connector is a Kubernetes operator that lets you manage GCP resources the same way you manage Kubernetes applications, and it’s a model that many other cloud platforms and infrastructure providers are adopting as well. Config Connector offers a compelling alternative to Terraform for managing IaC with a number of advantages.
First, because Config Connector is specific to GCP, it offers a more native integration with the platform. This includes more fine-grained status reporting that tells us the state of individual resources. It also lets us benefit from Kubernetes events for improved visibility. This allows us to provide greater visibility into what is happening with your infrastructure which results in a better overall developer experience.
Second, it enables us to use a combination of Kubernetes RBAC and GCP IAM for managing access control. With this model, we can have strong security boundaries and reduced blast radius as it relates to infrastructure.
Third, Config Connector provides an improved model for state management and reconciliation. With Terraform, managing state drift and environment promotions can be challenging. Additionally, the Terraform state file often contains secrets stored in clear text which is a security risk and requires the state file itself to be treated as highly sensitive data. Yet, Terraform does not support client-side state encryption but rather relies on at-rest encryption of the state backends (this is one of the areas Terraform and OpenTofu have diverged). It’s also common to hit race conditions in Terraform, where certain resources need to spin up before others can successfully apply. Sometimes this doesn’t happen and the deploy fails, requiring the apply to be re-run.
Config Connector takes a very different approach which solves these issues. Instead, it relies on a control loop which periodically reconciles resources to automatically correct drift. Think of it almost like “terraform apply” running regularly to ensure your desired state and actual state are in constant lockstep. The other benefit is it decouples dependent resources so we avoid race conditions and sequencing problems. This is possible because Config Connector models infrastructure as an eventually consistent state. This means resources can provision independently, even if their dependencies are not ready—no more re-running jobs to get a failed apply to work. Lastly, it can rely on Kubernetes secrets or GCP Secret Manager secrets to store sensitive information such as passwords or credentials. Sensitive data is properly isolated from our infrastructure configuration.
Finally, it’s possible to both bulk import existing resources into Config Connector and export resources to Terraform. This makes it possible to migrate existing infrastructure into Config Connector or migrate from Config Connector to Terraform. Config Connector can also reference resources that it does not manage using external references. This provides a means to gradually migrate to or from Config Connector.
Dealing with constraints and vendor lock-in
Konfig is different from typical PaaS offerings in that it really is an opinionated bundling of components rather than a proprietary, monolithic platform or “walled garden.” Normally, with PaaS systems like Google App Engine or Heroku, you relied on proprietary APIs to build applications which made it very difficult to migrate off when the time came. It also meant when you hit the limits of the platform, that’s it. There’s nothing you can do to work around them. At the other end of the spectrum are products like Crossplane, which are geared towards helping you build your own internal cloud platform. This puts you squarely back into the realm of staffing a team of highly paid platform engineers to build and maintain such a platform. For some companies, this might be a strategic place to invest. For most, it’s not.
And while there are proprietary value-add components to Konfig we have built, the core of it is products you’re already using—namely GitLab and GCP—and the open source Config Connector. The value of Konfig is that it is an opinionated implementation of these pieces that reduces an organization’s total cost of ownership for an enterprise cloud platform and shortens the delivery time for new software products. What this means, though, is that there really isn’t any vendor lock-in beyond whatever lock-in GitLab and GCP already have. Because it’s built around Config Connector, you can just as well use Config Connector to manage your resources directly, you’ll just lose the benefits of Konfig like GitLab and GCP integration and governance, automatic resource provisioning and IAM, ingress management, and the Konfig UI.
Config Connector provides us a powerful escape hatch, either in the case of needing to remove Konfig or needing to step outside Konfig’s opinions. If we want to remove Konfig, we have a couple options. We can export the Config Connector resource definitions that Konfig manages and import them into a new Config Connector instance. This Config Connector instance can be run either as a GKE add-on which is fully managed by Google, or it can be installed into a GKE cluster manually. Alternatively, we can export the resources managed by Konfig to Terraform.
If we need to step outside Konfig’s opinions, for example to provision a resource not currently supported by Konfig such as a VM, we can use Config Connector directly which supports a broad set of resources. We can manage these resources using the same GitOps model we use for Konfig workloads. With this, we have an opinionated platform that can support an organization’s most common needs but, unlike a PaaS, can grow and evolve with your organization.
Konfig reduces your cloud platform engineering costs and the time to deliver new software products. Reach out to learn more about Konfig or schedule a demo.
Follow @tyler_treat