Google Stackdriver provides a set of tools for monitoring and managing services running in GCP, AWS, or on-prem infrastructure. One feature Stackdriver has is “uptime checks,” which enable you to verify the availability of your service and track response latencies over time from up to six different geographic locations around the world. While Stackdriver uptime checks are not as feature-rich as other similar products such as Pingdom, they are also completely free. For GCP users, this provides a great starting point for quickly setting up health checks and alerting for your applications.
Last week I looked at implementing authentication and authorization for APIs in GCP using Cloud Identity-Aware Proxy (IAP). IAP provides an easy way to implement identity and access management (IAM) for applications and APIs in a centralized place. However, one thing you will bump into when using Stackdriver uptime checks in combination with IAP is authentication. For App Engine in particular, this can be a problem since there is no way to bypass IAP. All traffic, both internal and external to GCP, goes through it. Until Cloud IAM Conditions is released and generally available, there’s no way to—for example—open up a health-check endpoint with IAP.
While uptime checks have support for Basic HTTP authentication, there is no way to script more sophisticated request flows (e.g. to implement the OpenID Connect (OIDC) authentication flow for IAP-protected resources) or implement fine-grained IAM policies (as hinted at above, this is coming with IAP Context-Aware Access and IAM Conditions). So are we relegated to using Nagios or some other more complicated monitoring tool? Not necessarily. In this post, I’ll present a workaround solution for authenticating Stackdriver uptime checks for systems protected by IAP using Google Cloud Functions.
The Solution
The general strategy is to use a Cloud Function which can authenticate with IAP using a service account to proxy uptime checks to the application. Essentially, the proxy takes a request from a client, looks for a header containing a host, forwards the request that host after performing the necessary authentication, and then forwards the response back to the client. The general architecture of this is shown below.
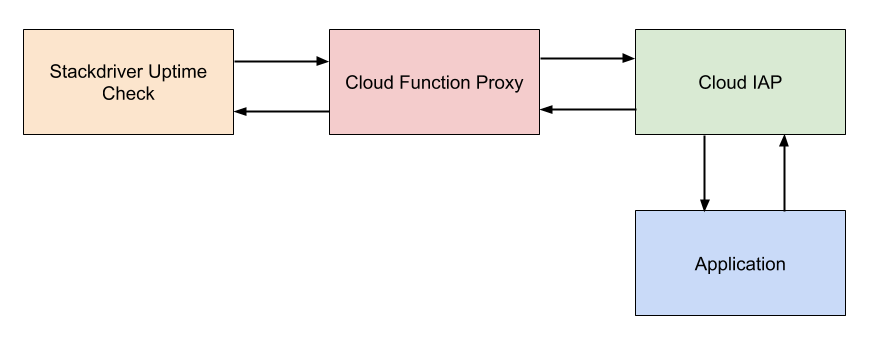
There are some trade-offs with this approach. The benefit is we get to rely on health checks that are fully managed by GCP and free of charge. Since Cloud Functions are also managed by GCP, there’s no operations involved beyond deploying the proxy and setting it up. The first two million invocations per month are free for Cloud Functions. If we have an uptime check running every five minutes from six different locations, that’s approximately 52,560 invocations per month. This means we could run roughly 38 different uptime checks without exceeding the free tier for invocations. In addition to invocations, the free tier offers 400,000 GB-seconds, 200,000 GHz-seconds of compute time and 5GB of Internet egress traffic per month. Using the GCP pricing calculator, we can estimate the cost for our uptime check. It generally won’t come close to exceeding the free tier.
The downside to this approach is the check is no longer validating availability from the perspective of an end user. Because the actual service request is originating from Google’s infrastructure by way of a Cloud Function as opposed to Stackdriver itself, it’s not quite the same as a true end-to-end check. That said, both Cloud Functions and App Engine rely on the same Google Front End (GFE) infrastructure, so as long as both the proxy and App Engine application are located in the same region, this is probably not all that important. Besides, for App Engine at least, the value of the uptime check is really more around performing a full-stack probe of the application and its dependencies than monitoring the health of Google’s own infrastructure. That is one of the goals behind using managed services after all. The bigger downside is that the latency reported by the uptime check no longer accurately represents the application. It can still be useful for monitoring aggregate trends nonetheless.
The Implementation Setup
I’ve built an open-source implementation of the proxy as a Cloud Function in Python called gcp-oidc-proxy. It’s runnable out of the box without any modification. We’ll assume you have an IAP-protected application you want to setup a Stackdriver uptime check for. To deploy the proxy Cloud Function, first clone the repository to your machine, then from there run the following gcloud command:
$ gcloud functions deploy gcp-oidc-proxy \
--runtime python37 \
--entry-point handle_request \
--trigger-http
This will deploy a new Cloud Function called gcp-oidc-proxy to your configured cloud project. It will assume the project’s default service account. Ordinarily, I would suggest creating a separate service account to limit scopes. This can be configured on the Cloud Function with the –service-account flag, which is under gcloud beta functions deploy at the time of this writing. We’ll omit this step for brevity however.
Next, we need to add the “Service Account Actor” IAM role to the Cloud Function’s service account since it will need it to sign JWTs (more on this later). In the GCP console, go to IAM & admin, locate the appropriate service account (in this case, the default service account), and add the respective role.
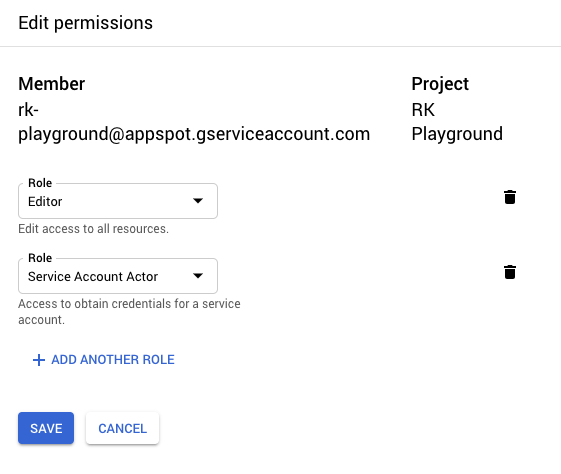
The Cloud Function’s service account must also be added as a member to the IAP with the “IAP-secured Web App User” role in order to properly authenticate. Navigate to Identity-Aware Proxy in the GCP console, select the resource you wish to add the service account to, then click Add Member.
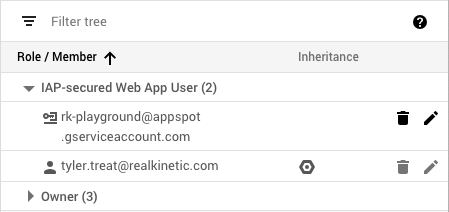
Find the OAuth2 client ID for the IAP by clicking on the options menu next to the IAP resource and select “Edit OAuth client.” Copy the client ID on the next page and then navigate to the newly deployed gcp-oidc-proxy Cloud Function. We need to configure a few environment variables, so click edit and then expand more at the bottom of the page. We’ll add four environment variables: CLIENT_ID, WHITELIST, AUTH_USERNAME, and AUTH_PASSWORD.
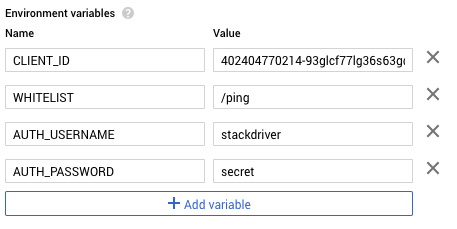
CLIENT_ID contains the OAuth2 client ID we copied for the IAP. WHITELIST contains a comma-separated list of URL paths to make accessible or * for everything (I’m using /ping in my example application), and AUTH_USERNAME and AUTH_PASSWORD setup Basic authentication for the Cloud Function. If these are omitted, authentication is disabled.
Save the changes to redeploy the function with the new environment variables. Next, we’ll setup a Stackdriver uptime check that uses the proxy to call our service. In the GCP console, navigate to Monitoring then Create Check from the Stackdriver UI. Skip any suggestions for creating a new uptime check. For the hostname, use the Cloud Function host. For the path, use /gcp-oidc/proxy/<your-endpoint>. The proxy will use the path to make a request to the protected resource.
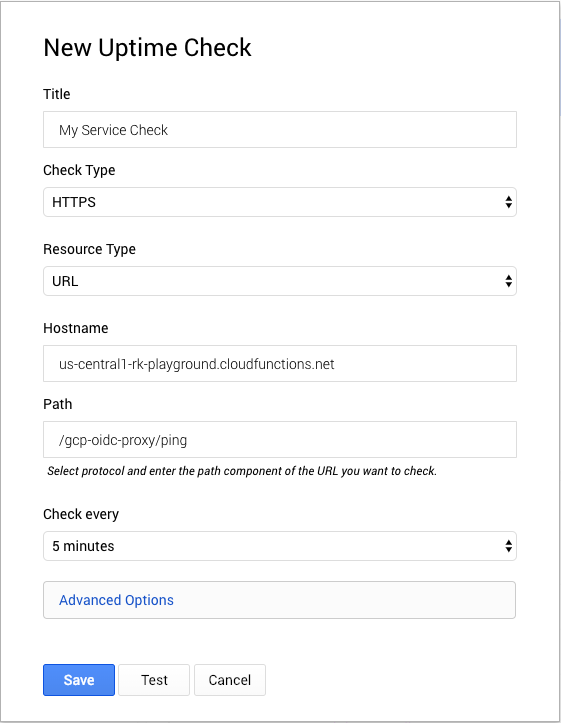
Expand Advanced Options to set the Forward-Host to the host protected by IAP. The proxy uses this header to forward requests. Lastly, we’ll set the authentication username and password that we configured on the Cloud Function.
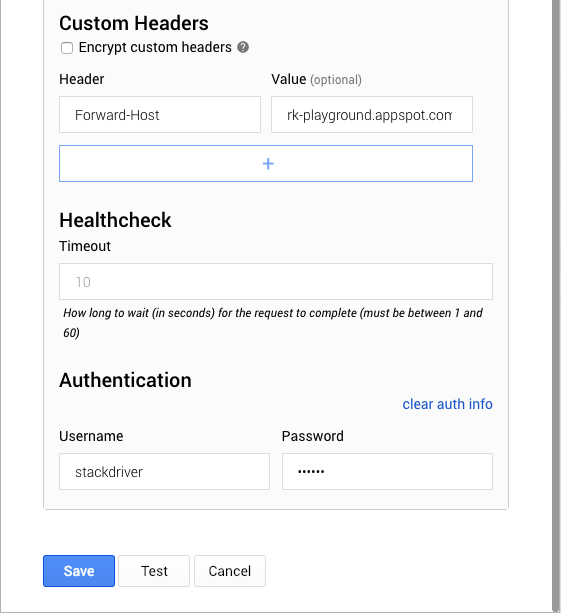
Click “Test” to ensure our configuration works and the check passes.
The Implementation Details
The remainder of this post will walk you through the implementation details of the proxy. The implementation closely resembles what we did to authenticate API consumers using a service account. We use a header called Forward-Host to allow the client to specify the IAP-authenticated host to forward requests to. If the header is not present, we just return a 400 error. We then use this host and the path of the original request to construct the proxy request and retain the HTTP method and headers (with the exception of the Host header, if present, since this can cause problems).
Before sending the request, we perform the authentication process by generating a JWT signed by the service account and exchange it for a Google-signed OIDC token.
We can cache this token and renew it only once it expires. Then we set the Authorization header with the OIDC token and send the request.
We simply forward on the resulting content body, status code, and headers. We strip HTTP/1.1 “hop-by-hop” headers since these are unsupported by WSGI and Python Cloud Functions rely on Flask. We also strip any Content-Encoding header since this can also cause problems.
Because this proxy allows clients to call into endpoints unauthenticated, we also implement a whitelist to expose only certain endpoints. The whitelist is a list of allowed paths passed in from an environment variable. Alternatively, we can whitelist * to allow all paths. Wildcarding could be implemented to make this even more flexible. We also implement a Basic auth decorator which is configured with environment variables since we can setup uptime checks with a username and password in Stackdriver.
The only other code worth looking at in detail is how we setup the service account credentials and IAM Signer. A Cloud Function has a service account attached to it which allows it to assume the roles of that account. Cloud Functions rely on the Google Compute Engine metadata server which stores service account information among other things. However, the metadata server doesn’t expose the service account key used to sign the JWT, so instead we must use the IAM signBlob API to sign JWTs.
Conclusion
It’s not a particularly simple solution, but it gets the job done. The setup of the Cloud Function could definitely be scripted as well. Once IAM Conditions is generally available, it should be possible to expose certain endpoints in a way that is accessible to Stackdriver without the need for the OIDC proxy. That said, it’s not clear if there is a way to implement uptime checks without exposing an endpoint at all since there is currently no way to assign a service account to a check. Ideally, we would be able to assign a service account and use that with IAP Context-Aware Access to allow the uptime check to access protected endpoints.